Making a license plate AI model
Results I have made so far
Handy things I have found along the way
This is a very rough written down experience of me trying to make my own model.
Getting the dataset
I simply hooked up a camera (Logitech C920) to my mac and recorded using OBS.
Preparing the dataset
First install label-studio. This application helps tremendously when making datasets!
pip install label-studio
label-studio
Once you’ve made your new project (click on Create on the top right) and have imported the data, you are greeted with this:
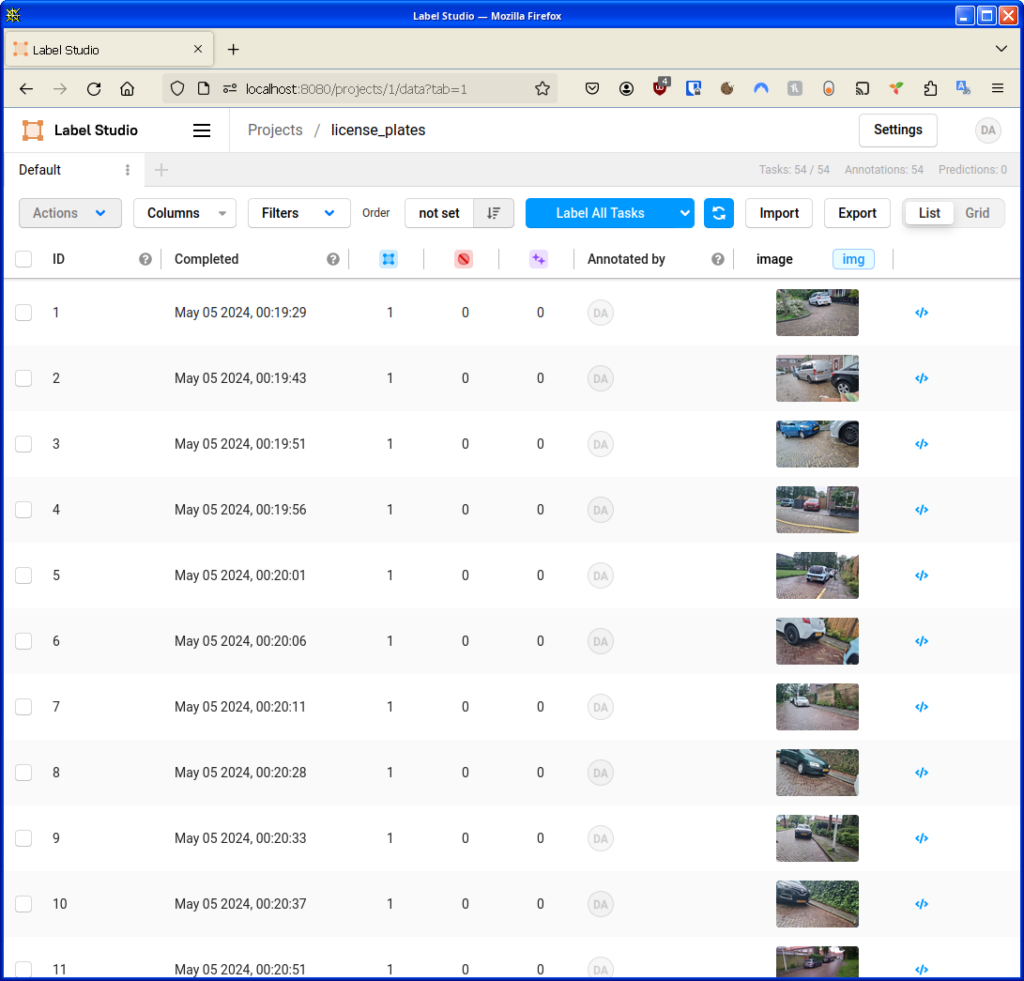
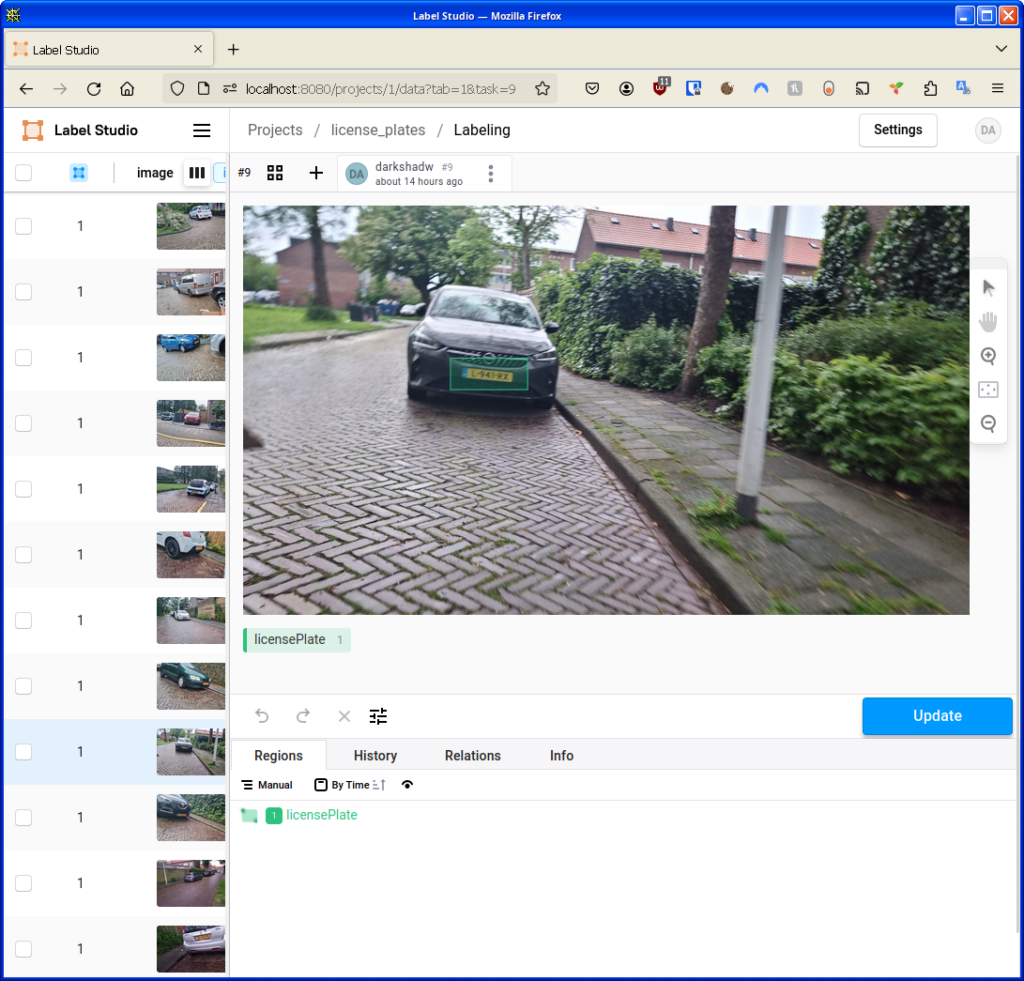
After tagging your images, export it to pascal.
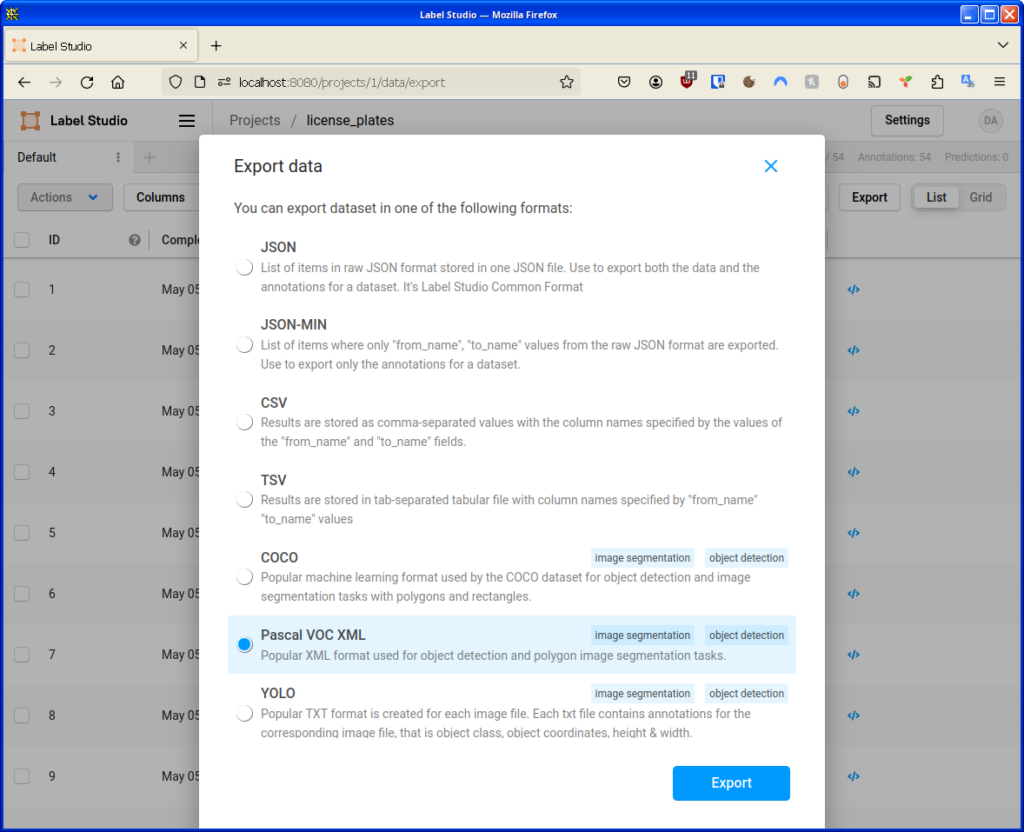
Extract the export to dataset/licensePlate/pascal_data
Now convert the Pascal export to a CSV using prepare/xml_to_csv.py
like so:
python prepare/xml_to_csv.py --annot_dir dataset/licensePlate/pascal_data/Annotations/ --out_csv_path dataset/licensePlate/train_labels.csv
First make a file in dataset/licensePlate
called license_plate.pbtxt
with the following content:
item {
id: 1
name: 'licensePlate'
display_name: 'License Plate'
}
item {
id: 2
name: 'car'
display_name: 'Car'
}
Now convert the csv into a tfrecord:
python prepare/generate_tfrecord.py --path_to_images dataset/licensePlate/pascal_data/images --path_to_annot dataset/licensePlate/train_labels.csv --path_to_label_map dataset/licensePlate/license_plate.pbtxt --path_to_save_tfrecords dataset/licensePlate/train.record
Edit the train.config next. My file as of right now is:
# SSD with Mobilenet v2
# Trained on COCO17, initialized from Imagenet classification checkpoint
# Train on TPU-8
#
# Achieves 22.2 mAP on COCO17 Val
model {
ssd {
inplace_batchnorm_update: true
freeze_batchnorm: false
num_classes: 2
box_coder {
faster_rcnn_box_coder {
y_scale: 10.0
x_scale: 10.0
height_scale: 5.0
width_scale: 5.0
}
}
matcher {
argmax_matcher {
matched_threshold: 0.5
unmatched_threshold: 0.5
ignore_thresholds: false
negatives_lower_than_unmatched: true
force_match_for_each_row: true
use_matmul_gather: true
}
}
similarity_calculator {
iou_similarity {
}
}
encode_background_as_zeros: true
anchor_generator {
ssd_anchor_generator {
num_layers: 6
min_scale: 0.2
max_scale: 0.95
aspect_ratios: 1.0
aspect_ratios: 2.0
aspect_ratios: 0.5
aspect_ratios: 3.0
aspect_ratios: 4.73
aspect_ratios: 0.3333
}
}
image_resizer {
fixed_shape_resizer {
height: 300
width: 300
}
}
box_predictor {
convolutional_box_predictor {
min_depth: 0
max_depth: 0
num_layers_before_predictor: 0
use_dropout: false
dropout_keep_probability: 0.8
kernel_size: 1
box_code_size: 4
apply_sigmoid_to_scores: false
class_prediction_bias_init: -4.6
conv_hyperparams {
activation: RELU_6,
regularizer {
l2_regularizer {
weight: 0.00004
}
}
initializer {
random_normal_initializer {
stddev: 0.01
mean: 0.0
}
}
batch_norm {
train: true,
scale: true,
center: true,
decay: 0.97,
epsilon: 0.001,
}
}
}
}
feature_extractor {
type: 'ssd_mobilenet_v2_keras'
min_depth: 16
depth_multiplier: 1.0
conv_hyperparams {
activation: RELU_6,
regularizer {
l2_regularizer {
weight: 0.00004
}
}
initializer {
truncated_normal_initializer {
stddev: 0.03
mean: 0.0
}
}
batch_norm {
train: true,
scale: true,
center: true,
decay: 0.97,
epsilon: 0.001,
}
}
override_base_feature_extractor_hyperparams: true
}
loss {
classification_loss {
weighted_sigmoid_focal {
alpha: 0.75,
gamma: 2.0
}
}
localization_loss {
weighted_smooth_l1 {
delta: 1.0
}
}
classification_weight: 1.0
localization_weight: 1.0
}
normalize_loss_by_num_matches: true
normalize_loc_loss_by_codesize: true
post_processing {
batch_non_max_suppression {
score_threshold: 1e-8
iou_threshold: 0.6
max_detections_per_class: 100
max_total_detections: 100
}
score_converter: SIGMOID
}
}
}
train_config: {
fine_tune_checkpoint_version: V2
fine_tune_checkpoint: "models/ssd_mobilenet_v2_320x320_coco17_tpu-8/checkpoint/ckpt-0"
fine_tune_checkpoint_type: "detection"
batch_size: 64
sync_replicas: true
startup_delay_steps: 0
replicas_to_aggregate: 8
num_steps: 20000
data_augmentation_options {
random_horizontal_flip {
}
}
data_augmentation_options {
ssd_random_crop {
}
}
optimizer {
momentum_optimizer: {
learning_rate: {
cosine_decay_learning_rate {
learning_rate_base: 0.0004
total_steps: 20000
warmup_learning_rate: 0.0004
warmup_steps: 2000
}
}
momentum_optimizer_value: 0.9
}
use_moving_average: false
}
max_number_of_boxes: 26
unpad_groundtruth_tensors: false
}
train_input_reader: {
label_map_path: "dataset/licensePlate/license_plate.pbtxt"
tf_record_input_reader {
input_path: "dataset/licensePlate/train.record"
}
}
eval_config: {
metrics_set: "coco_detection_metrics"
use_moving_averages: false
}
eval_input_reader: {
label_map_path: "dataset/licensePlate/license_plate.pbtxt"
shuffle: false
num_epochs: 1
tf_record_input_reader {
input_path: "dataset/licensePlate/train.record"
}
}
Training the model
I have edited some files:
out_dir=models/licensePlate/
mkdir -p $out_dir
python training/model_main_tf2.py --alsologtostderr --model_dir=$out_dir --checkpoint_every_n=500 --pipeline_config_path=dataset/licensePlate/train.config --eval_on_train_data 2>&1 | tee $out_dir/train.log
model_dir=models/licensePlate/
out_dir=$model_dir/exported_model
mkdir -p $out_dir
python exporter_main_v2.py \
--input_type="image_tensor" \
--pipeline_config_path=$model_dir/pipeline.config \
--trained_checkpoint_dir=$model_dir/ \
--output_directory=$out_dir
python test_model/detect_objects.py --video_input --threshold 0.5 --model_path models/licensePlate/exported_model/saved_model --path_to_labelmap dataset/licensePlate/license_plate.pbtxt --video_path
Filed under: AI,Object Recognition - @ May 4, 2024 4:55 pm